Can Ai help solve the biggest challenges with CSAT surveys?
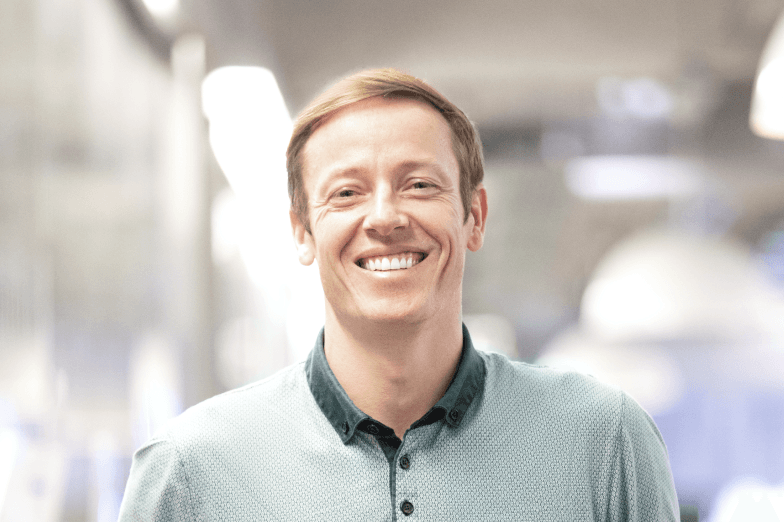
Chief Ai & Strategy Officer
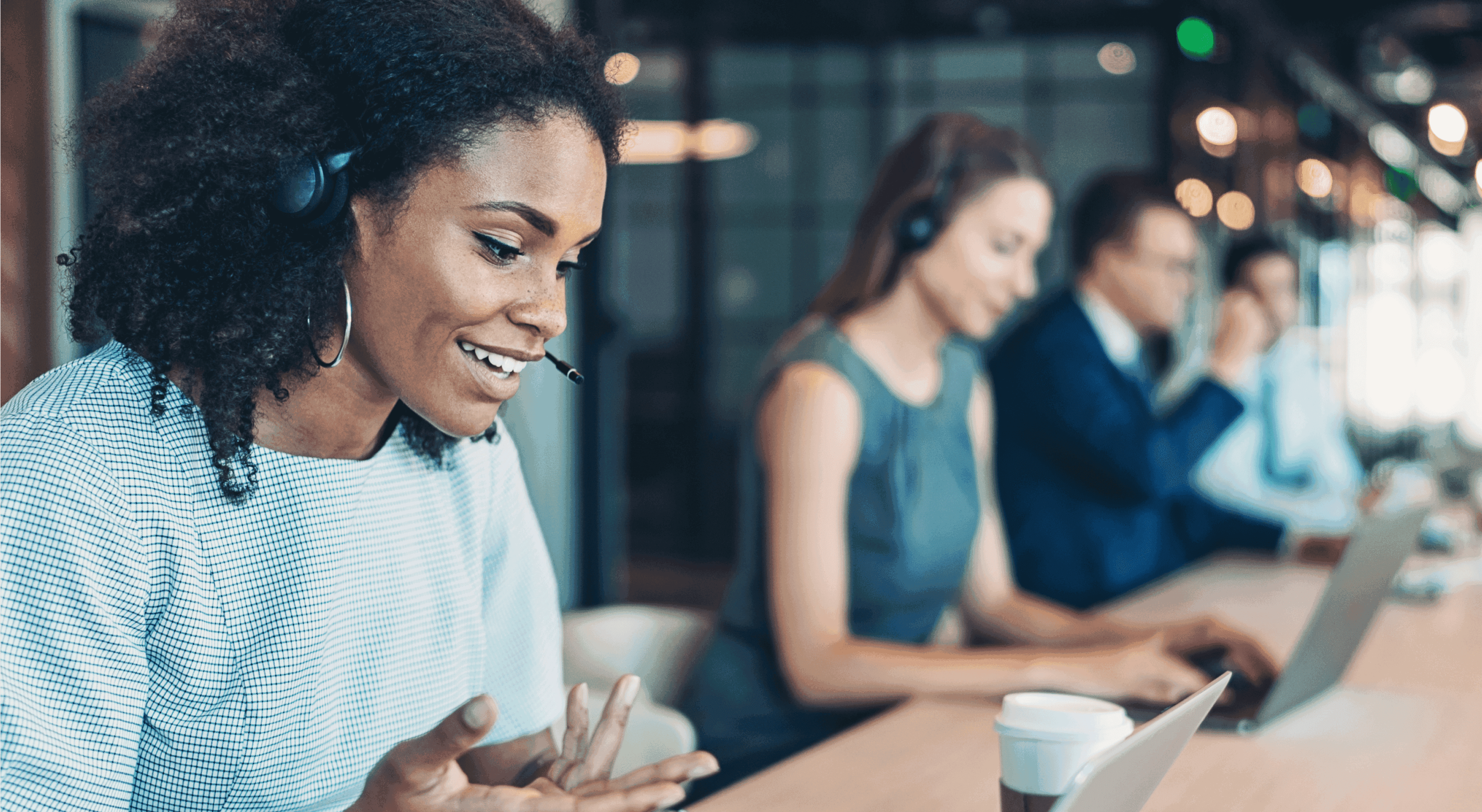
Tags
Share
Think about the last time you called a customer support number for help. At the end of the conversation, were you asked to fill out a survey that rates your satisfaction?
Did you fill it out?
Let’s be honest, most people end the call before getting to the survey. According to our analysis at Dialpad, the response rate of customer satisfaction (CSAT) surveys is only 3%.
Low CSAT survey response rates impact businesses in a couple of ways:
Shows just the tip of the iceberg: Traditional CSAT surveys are often biased because respondents provide either extremely positive or negative responses—meaning you’re missing out on the chunk of insights from customers who were “just” content or satisfied.In addition, with low numbers of CSAT responses, there can be large upswings and downswings in daily CSAT for any time period. This makes it difficult for contact center managers to monitor changes in CSAT scores at any given time. This can cause insights to be unreliable, making the data less effective.
Missed follow-up and coaching opportunities: Contact center managers lack the ability to be proactive in following up with customers that have low customer satisfaction but don’t fill out a survey. It can also be difficult for coaches to find the best agent coaching opportunities because of the lack of call data.
To help solve these issues, we built an Ai model that predicts customer satisfaction—even when they don’t fill out a CSAT survey.
We call it Ai CSAT.
Ai CSAT: How we built it
The Ai model that we use is a large, state-of-the-art neural network trained on proprietary data. Our model uses call transcriptions to predict CSAT. These call transcriptions are generated by Dialpad’s own speech transcription technology (which has the highest accuracy compared to other speech transcription services), which has been crucial in our model’s success to predict CSAT reliably. As explained below, the model undergoes a pre-training phase and a training phase to achieve best performance.
You may ask: “Why is training important?”
The process of training this model is similar to how kids learn a new language—that is, by training on a large amount of data by speaking and reading. Similar to how a brain changes with learning, our Ai model constantly updates its 100+ million parameters in response to training data.
Walking before crawling: Pre-training an Ai to read before predicting CSAT
Before training the model on predicting CSAT, it’s helpful to pre-train the model. This helps the model understand how English and call center conversations work. Specifically, we pre-trained the model on a proprietary dataset of 1.28 million call center conversations. During this phase, the model is given a sliding window of words as context and continuously tries to predict the next word in the conversation. This is similar to how babies listen and learn to spot patterns in language even before they start forming words.
Training the model to predict CSAT
In the main training phase, we presented the model with pairs of transcripts + CSAT survey responses. The task in this phase is to predict the CSAT given the call transcript:
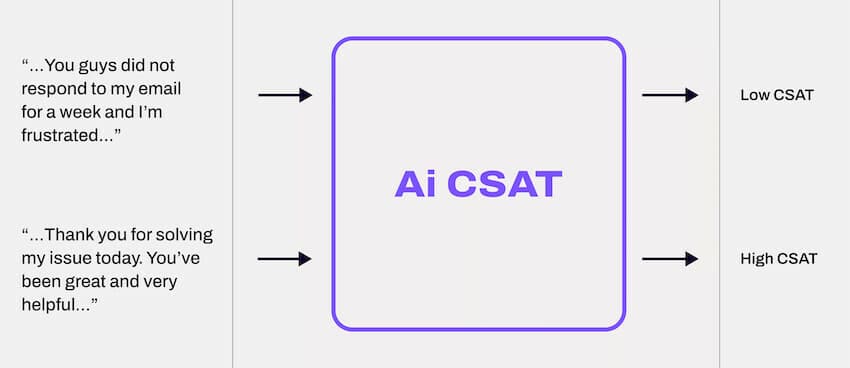
During the training process, the model updates its millions of parameters to maximize its accuracy.
Reducing bias: How we achieved an accurate Ai CSAT model
Now, here’s a question for you: Is it fair for a contact center manager to use CSAT responses as a measure of how well a contact center is performing, knowing that only 3% of calls have a CSAT response?
The short answer is NO!
The reason for that is, what if the remaining 97% who didn’t respond all had a positive experience and saw no need to leave feedback? In this case, there’s a response bias, which means we cannot draw any insights or definitive conclusion from that CSAT survey.
As an example, in one contact center, we saw that the average CSAT score from survey responses was 24% lower than the average Ai CSAT score that was generated by the model.
But wait—is it the Ai CSAT model that’s overestimating customer satisfaction, or could it be that dissatisfied customers leave survey responses at a higher rate, artificially lowering the CSAT score?
To distinguish between these hypotheses, we took the Ai CSAT scores that we generated for a set of calls and compared them with the actual CSAT scores from the survey responses to see if they matched up.
Our discovery: The Ai CSAT score was almost equal to the CSAT score!
This meant that it’s not the model that is biased, but rather that there’s response bias in the survey. In other words, customers that had a negative experience took the survey at a higher rate than those with a positive experience. Without Ai CSAT scores, we could not have uncovered this insight!
Through this rigorous training and testing process, we managed to develop Ai CSAT to be hyper-accurate. We were also able to 20x the number of calls associated with CSAT scores. That 3% response rate I mentioned earlier went up to 70%.
👉 SIDE NOTE:
To make sure our Ai maintained accuracy as it predicted CSAT scores, we stress-tested the model against different scenarios. For example, in one experiment, we observed that both female and male customer experience agents have similar average Ai CSAT scores, meaning the model didn’t over-predict scores for one or the other and does not display gender bias.
How Ai CSAT scores make learnings more comprehensive and reliable
Last but not least, let’s look at a specific contact center as an example in the graph below. The orange line shows the (more limited) number of customer-collected CSAT survey responses and as you can see, there are big daily swings (high variance):
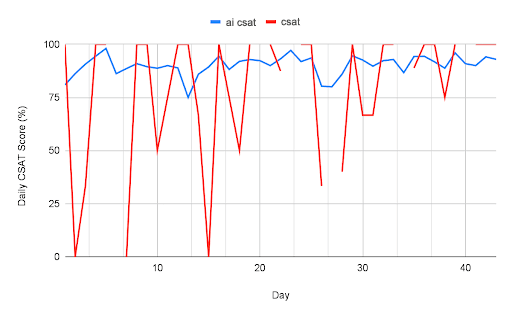
Looking at this sparse data, a manager might worry about the three very low CSAT ratings starting on Day 2—but they can’t be sure if this is part of an actual trend because the sample size is so small.
Now, let’s look at the blue line which shows our Ai CSAT scores. It’s far more stable day-over-day because we have a much larger amount of data to draw from, and actually, we can see a modest upward trend (~2%) in scores over the time period.
With this data in hand, the contact center manager knows now that they shouldn’t overreact too quickly to a handful of low CSAT ratings from customers. Now, they can see more data and have a more representative sample size of calls.
Get more actionable and reliable insights with Dialpad’s Ai CSAT
There’s a vast range of uses for Ai technology, and the possibilities are literally endless, especially if you are building your own Ai, as we are here at Dialpad.
We’re excited that Dialpad’s Ai is now mature enough to predict CSAT with high accuracy while helping solve some of CSAT surveys’ biggest issues—low response rate and response bias.
With ongoing improvements and machine learning, this model will only get more accurate over time and continue to help contact center managers understand their customers’ satisfaction on a much more holistic level.
Stay tuned for more exciting developments from our Ai team!
See Ai CSAT in action!
Take a product tour of Dialpad Ai Contact Center!